commit | 3cbdaf04b44c1d65f2d4e06f2d5d3a103e76573a | [log] [tgz] |
---|---|---|
author | Heres, Daniel <danielheres@gmail.com> | Wed Apr 07 12:44:04 2021 -0400 |
committer | Andrew Lamb <andrew@nerdnetworks.org> | Wed Apr 07 12:44:04 2021 -0400 |
tree | a4e95271da22afae2be206b521321a1de3d1f413 | |
parent | 10f3f9579adf5fa53def8e586d1082f378bf4fc7 [diff] |
ARROW-12190: [Rust][DataFusion] Implement parallel / partitioned hash join This implements a first version of parallel / partitioned hash join / grace join and uses it by default in the planner (for concurrency > 1) Master (in memory / 16 threads / partitions, SF=1, batch size = 8192) ``` Query 5 avg time: 141.29 ms Query 10 avg time: 477.63 ms ``` PR ``` Query 5 avg time: 91.60 ms Query 10 avg time: 272.25 ms ``` Also when loading from parquet it also achieves ~20% speed up in total and has better utilization of all threads (this is SF=10 with 16 partitions on 16 threads in Parquet running 10 iterations): 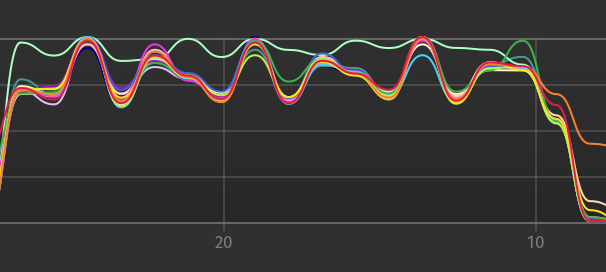 FYI @andygrove Note: I think the same approach could also be used by the hash aggregate, rather than the partial / full approach taken now, but this might be only be better when we have a high number of distinct group by by values (in extreme cases - groups with one row), e.g. the "full" hash aggregate takes up a lot of the time/memory. @alamb I think your earlier comment w.r.t. "CoalesceBatches" is especially applicable here - I am seeing quite some overhead in CoalesceBatches/Concat after `RepartitionExec` with hash repartitioning, as this will make each produced batch roughly `num_partitions` times smaller (in contrast to roundrobinbatch repartitioning - which keeps the same batches which makes it almost free on a single node). The overhead is so big that lowering the concurrency setting to 8 instead of 16 on my 8-core / 16-hyperthreads laptop yields better performance. Increasing the batch size (to 32000-64000) also recovers the performance / avoids the overhead penalty mostly - but I recently reduced this as for most queries ~8000 was the sweet spot. Rewriting to buffer them inside the `RepartitionExec` and only produce them when they exceed the batch size probably would solve this issue and improve the performance of the parallel hash join quite a bit - or maybe by optimizing the `concat` kernel. Closes #9882 from Dandandan/grace_join Lead-authored-by: Heres, Daniel <danielheres@gmail.com> Co-authored-by: Daniƫl Heres <danielheres@gmail.com> Signed-off-by: Andrew Lamb <andrew@nerdnetworks.org>
Apache Arrow is a development platform for in-memory analytics. It contains a set of technologies that enable big data systems to process and move data fast.
Major components of the project include:
Arrow is an Apache Software Foundation project. Learn more at arrow.apache.org.
The reference Arrow libraries contain many distinct software components:
The official Arrow libraries in this repository are in different stages of implementing the Arrow format and related features. See our current feature matrix on git master.
Please read our latest project contribution guide.
Even if you do not plan to contribute to Apache Arrow itself or Arrow integrations in other projects, we'd be happy to have you involved: